
This data was still found to have occasional un-labeled buildings, label shifting, and patches of no data that cause the labels to not be usable until edited. Tier 1 data sets represent urban extents of Ghana, Uganda, Republic of Congo, and Tanzania and is higher in quality in comparison to Tier 2. The data is split between two tiers: Tier 1 and Tier 2 Consult the ramp data dictionary to identify the datasets that have already been used in the baseline model (avoid these for fine tuning). These datasets would be helpful for fine tuning the ramp model over similar African urban geographies but would not be ideal otherwise. The imagery behind these sources are also covered in more detail in the Imagery section. Note that re-exposing a fine-tuned model to data that it has already ingested in the baseline model will not work to tune the model any further. What follows is a brief overview of a few datasets that we used in training the baseline model and could prove beneficial in fine-tuning as well. Utilizing existing datasets also limits the geographic extent of the data, which is an important consideration when fine tuning the ramp model. The review and editing process will be highlighted in a subsequent section. It can also become time consuming when reviewing and editing the labels to match ramp specifications. That being said, you have no control over the way the dataset was created which can pose issues when the labeling style or image resolution conflicts with the specifications that have been followed to train the ramp baseline model. Of the three options highlighted in this documentation, this is the cheapest and potentially least time-consuming path to acquiring the training data needed. The closest a labeler is able to follow these guidelines the better as the baseline ramp model has learned to recognize the patterns from this specific label style, and will preform better when fine-tuned with the same approach.Īnother feasible way to acquire the training data needed for fine-tune training the ramp model is to find training data that has already been created and has a favorable open source license. The ramp team has put together a label guidance document that was utilized to train the ramp baseline ramp model and a few of it’s early fine-tuned models. The more the labeling process can be mimicked from one labeler to another, the better. This is important when a team of labelers is working on labeling, as each person will have their own style. Consistency is key, and all labels created should be done so in the same format and to the same specifications. Label creation can become tedious and time consuming, but is a critical step in the process and will have a large impact on the model accuracy. When creating your own training data, you are responsible for creating the building labels in each image tile. The high resolution satellite imagery serves as the foundation of the training data, but is useless without building labels (vector polygon labels) overlaying the imagery. When selecting your imagery for training, make sure to give it a good look over and review for overall quality, considering cloud cover, image tilt or off nadir angle (should be less than 30 degrees), spatial resolution (size of the pixel (which should be between 30-50cm for the ramp model), and temporal resolution (when was the imagery collected? Does age impact usability for the use case?). For more detail on Imagery sources see the previous Imagery section.
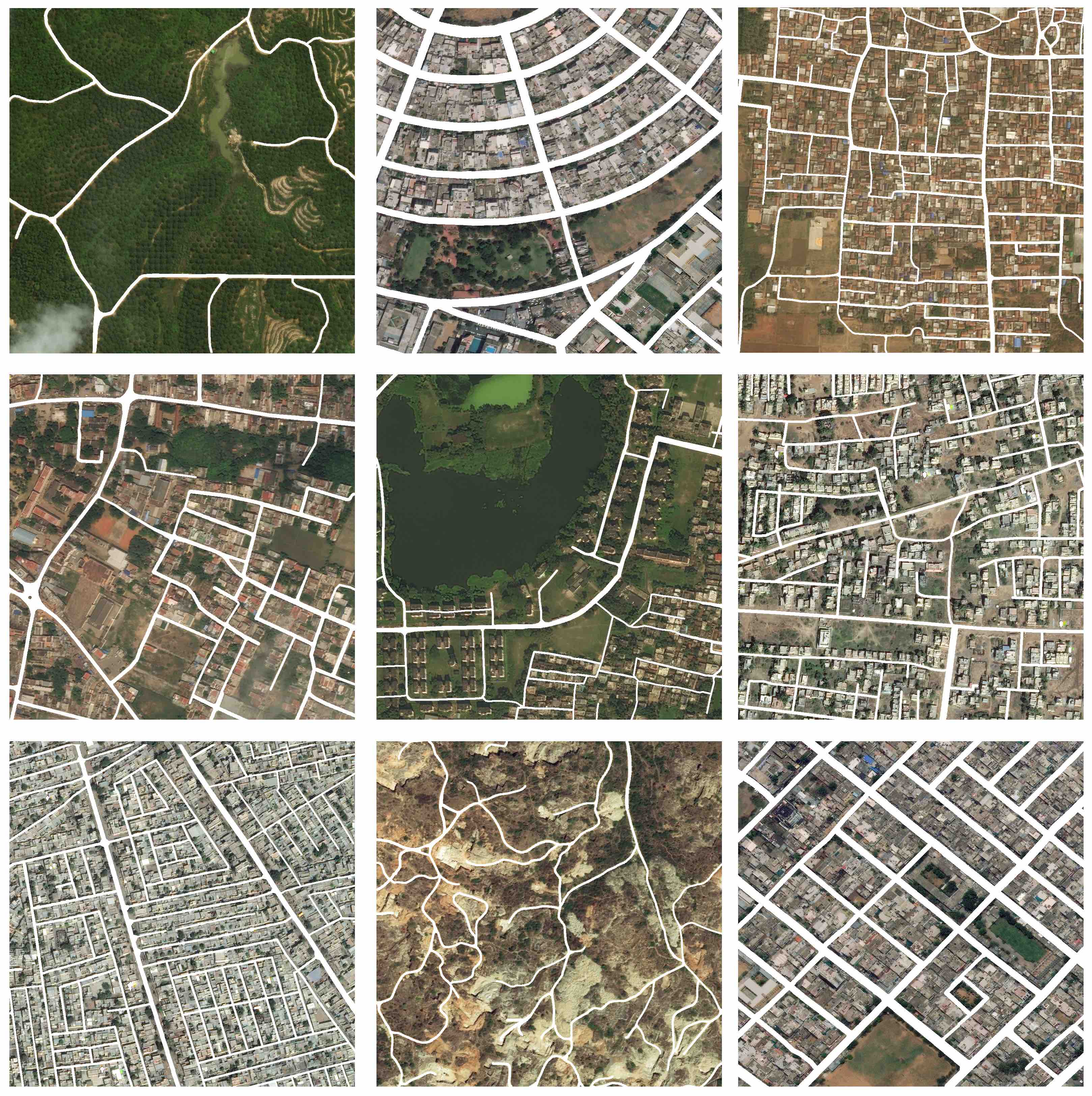
There are multiple sources of satellite imagery out there (Maxar Secure Watch, Planet, list a few of them), and selecting your source of imagery depends on various factors including budget and timeframe. In creating your own training data, you have the most control over the quality of the data and can tailor the data exactly to your needs.Īcquiring Imagery is the first step in the training data process. Your task is to acquire satellite imagery and create the building outline vector labels on the imagery, which will be used together to train the ramp model.
